The rise of ‘exascale’ and ‘zettascale’ supercomputing has transformed discussions around AI performance, but are these claims valid? In this article, we explore how AI systems perform, contrasting hype with verified results. Expert Doug Eadline reveals how inflated metrics can mislead, underscoring why trusted benchmarks matter. Learn what sets HPC and AI FLOPS apart in the race toward true zettascale capabilities.
When it comes to supercomputing, terms like “exascale” and “zettascale” have become buzzwords in the tech world. Yet, despite widespread use, experts question the true validity of these claims, especially about AI workloads. Doug Eadline from HPCWire, a leading figure in high-performance computing (HPC), recently unpacked the technical misunderstandings around these terms. His insights raise important questions about whether AI systems today genuinely achieve the performance levels implied by “exascale” or “zettascale” labels.
Understanding Exascale and Zettascale: What Do They Mean?
In the traditional HPC context, “exascale” refers to a computer’s ability to perform one quintillion floating-point operations per second (FLOPS) in double-precision (64-bit) calculations. This is an astronomical feat, achievable only through rigorous benchmarking, particularly with tests like the High-Performance LINPACK (HPLinpack) benchmark, which validates true exascale capabilities. When used accurately, terms like “exascale” or “zettascale” indicate computing systems with exceptionally high, sustained performance, not speculative or peak metrics.
Yet, as Eadline emphasizes, many claims of AI systems achieving zettascale or exascale status rest on theoretical numbers. Often, these figures are based on a system’s potential rather than its verified performance. “How do these ‘snort your coffee’ numbers arise from unbuilt systems?” he questions, highlighting how these inflated metrics often fail to reflect actual, operational performance.
AI FLOPS vs. HPC FLOPS: The Importance of Precision
A fundamental difference exists between AI-focused FLOPS and those in traditional HPC. AI workloads tend to use lower-precision floating-point formats like FP16, FP8, or even FP4, which are sufficient for tasks like image recognition or natural language processing. However, higher precision—specifically double-precision FP64—is essential in HPC to maintain accuracy in complex simulations and scientific computations.
The use of lower-precision numbers in AI workloads has led to exaggerated claims of exaFLOP or even zettaFLOP performance. Eadline points out, “Calling it ‘AI zetaFLOPS’ is silly because no AI was run on this unfinished machine.” By relying on lower-precision calculations, these claims overlook the stringent requirements necessary for a system to genuinely qualify as exascale or zettascale in the HPC sense.
Did You Know?
Exascale computers require sustained, verified performance levels, often achieved through benchmarks like HPLinpack, which has been the standard in HPC since 1993.
A Car Analogy: Explaining the Difference in Precision
Eadline uses an accessible car analogy to illustrate the variance between AI and HPC FLOPS. Think of a traditional double-precision (FP64) vehicle as a fully equipped, high-performing car—an SUV that comfortably carries passengers across diverse terrains, maintaining a reasonable fuel economy. In contrast, the FP4 vehicle, representing lower-precision AI systems, is more like a stripped-down scooter that achieves staggering mileage but lacks essentials.
With AI-focused FLOPS:
- FP64 Car: Weighs about 4,000 pounds (1,814 kg), navigates well, and offers a smooth ride on various terrains.
- FP4 Scooter: Weighs only 250 pounds (113 kg), and provides 480 MPG, but lacks basic comforts and delivers a much bumpier ride.
While the FP4 “car” might be efficient in specific scenarios, it falls short of delivering the comprehensive, versatile performance of the FP64 vehicle. Eadline’s analogy underscores that while AI systems may seem impressive in benchmarks, they often lack the robustness required for high-stakes scientific computing in the HPC realm.
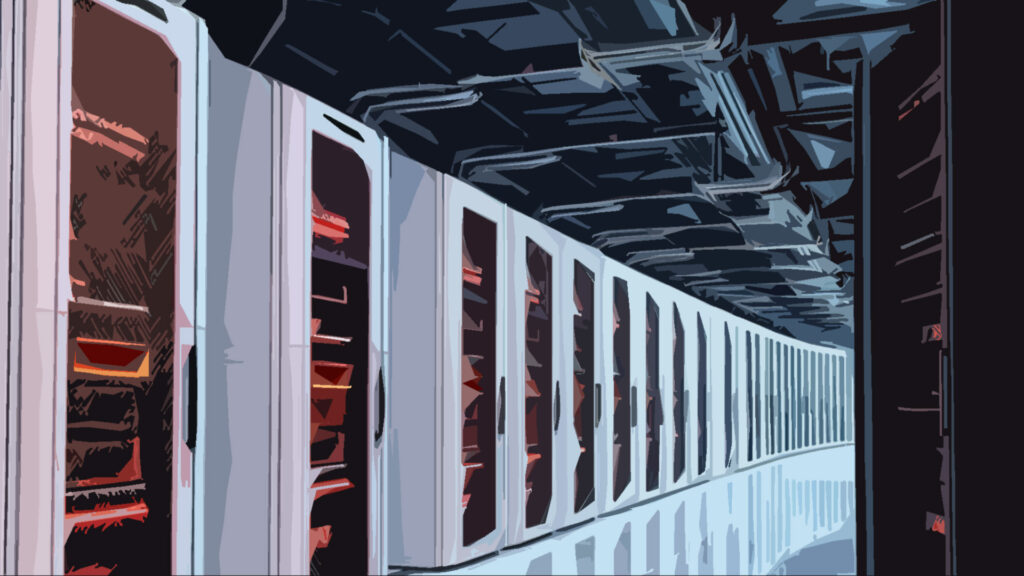
The Real Exascale Machines: Frontier and Aurora
Only two supercomputers—Frontier at Oak Ridge National Laboratory and Aurora at Argonne National Laboratory—are recognized as achieving true exascale status. Unlike many AI systems making exascale claims, these systems have been rigorously tested using double-precision calculations and validated benchmarks. Unlike the speculative metrics often attached to AI-focused machines, Frontier and Aurora have demonstrated real, measurable results.
Why Misrepresenting FLOPS Matters
Misleading performance metrics pose real issues for the tech community. When companies present theoretical, peak values as representative of a system’s true capabilities, they contribute to unrealistic expectations. This confusion can mislead investors, misallocate resources, and ultimately hinder advancements in both AI and HPC fields. Eadline’s article serves as a reminder that using accurate, verified benchmarks—not speculative numbers—benefits everyone in the high-performance computing ecosystem.
Why Verified Benchmarks Matter in HPC and AI Convergence
As AI and HPC continue to converge, setting clear, accurate standards for performance measurement is essential. Verified benchmarks, such as HPLinpack, ensure that systems meet the rigorous demands of scientific computing. Only those that pass these tests should be granted exascale or zettascale designations. “Fuzzing things up with ‘AI FLOPS’ will not help either,” Eadline remarks, advocating for transparency and accuracy in supercomputing benchmarks.
Key Takeaways
- Exascale and zettascale labels should only apply to systems meeting verified, high-precision performance standards—not speculative figures.
- AI FLOPS and HPC FLOPS differ greatly: AI workloads can rely on lower-precision formats, which inflates performance numbers without necessarily meeting HPC’s rigorous requirements.
- Transparent benchmarks like HPLinpack provide a consistent standard that differentiates between peak theoretical performance and verified sustained output.
- Inflated AI performance claims may mislead stakeholders and potentially stall development by creating unrealistic expectations.